
The probability density and cumulative distribution functions may be given using PDF, x ] and CDF, x ].Such an assertion can then be used in functions such as Probability, NProbability, Expectation, and NExpectation.

Distributed ], written more concisely as x NormalDistribution, can be used to assert that a random variable x is distributed according to a normal distribution. RandomVariate can be used to give one or more machine- or arbitrary-precision (the latter via the WorkingPrecision option) pseudorandom variates from a normal distribution.
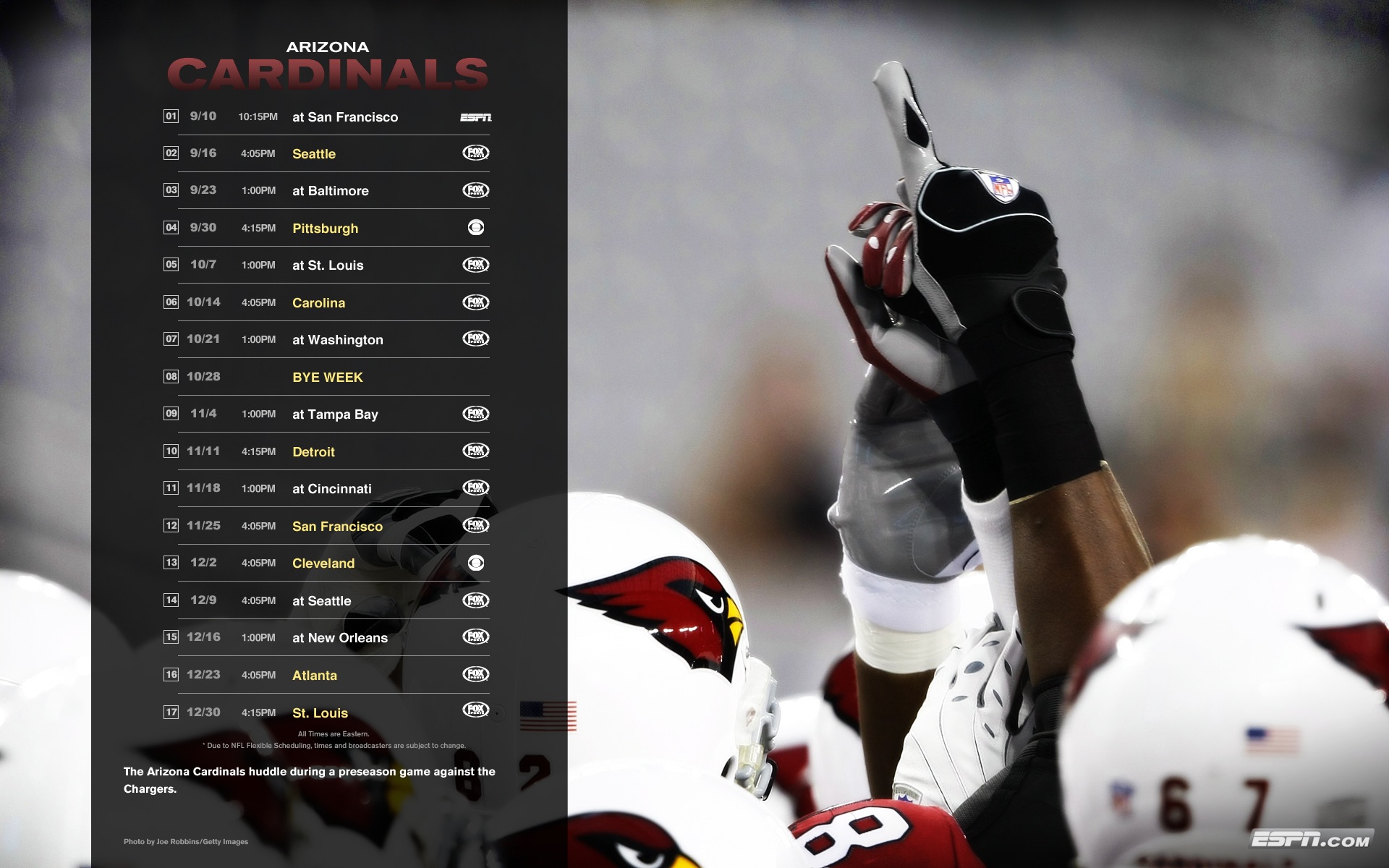
In addition, a large number of biological phenomena, including sizes of living tissue and quantities such as fasting blood glucose level and blood pressure, yield variables whose logarithms tend to be normally distributed. The normal distribution also arises naturally when modeling a number of physical phenomena, such as the velocity of ideal gas molecules, the positions of particles experiencing diffusion, and the long-timescale behavior of thermal light. Furthermore, because of the central limit theorem, the mean of a sufficiently large number of independent random variables will be approximately normally distributed provided certain hypotheses are satisfied, regardless of the original distributions describing the variables. Moreover, a number of probabilistic and statistical values including percentile ranks and - and -scores are derived from normal distributions. In addition, the normal distribution is also fundamental in defining the so-called Wiener process, a continuous-time stochastic process consisting of independent increments, each of which is independent and identically normally distributed with and for. For example, normally distributed values are of fundamental importance in applications of the Monte Carlo method.
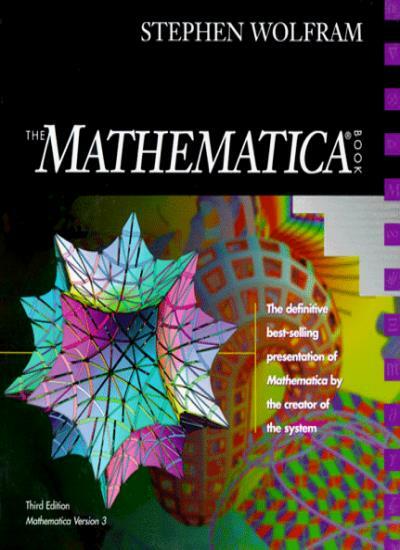
(This behavior can be made quantitatively precise by analyzing the SurvivalFunction of the distribution.) The zero-argument form NormalDistribution is equivalent to NormalDistribution and is sometimes called the standard normal distribution.
MATHEMATICA 7 PRICE PDF
The PDF of a normal distribution is symmetric about its maximum, and the tails of its PDF are "thin" in the sense that the PDF decreases exponentially for large values of. The probability density function (PDF) of a normal distribution is unimodal, with the peak occurring at the mean, and the parameter σ determines both the height of the PDF and the "thickness" of its tails.

The distribution is parametrized by a real number μ and a positive real number σ, where μ is the mean of the distribution, σ is known as the standard deviation, and σ 2 is known as the variance.
